Seeded watershed for membrane-based cell segmentation
Contents
Seeded watershed for membrane-based cell segmentation#
In this section we will use a seeded watershed approach to cell segmentation. This approach is very common when cell segmentation based on images of membrane markers are given. Therefore, we use the napari plugin napari-segment-blobs-and-things-with-membranes. Under the hood, this plugins uses functions from scikit-image.
See also
from napari_segment_blobs_and_things_with_membranes import voronoi_otsu_labeling, \
seeded_watershed, \
local_minima_seeded_watershed
from skimage.io import imread
from skimage.filters import gaussian
from skimage import data
from pyclesperanto_prototype import imshow
We load the Cells3d example image from scikit-image which is a two-channel image showing nuclei and membranes.
cells = data.cells3d()
cells.shape
(60, 2, 256, 256)
nuclei_channel = cells[30, 1]
imshow(nuclei_channel)
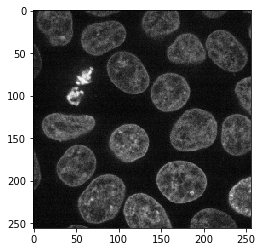
membrane_channel = cells[30, 0]
imshow(membrane_channel, max_display_intensity=6000)
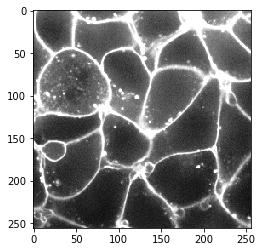
Voronoi-Otsu-Labeling for nuclei segmentation#
First, we start with segmenting the nuclei using the Voronoi-Otsu-Labeling algorithm.
labeled_nuclei = voronoi_otsu_labeling(nuclei_channel, spot_sigma=10, outline_sigma=2)
imshow(labeled_nuclei, labels=True)
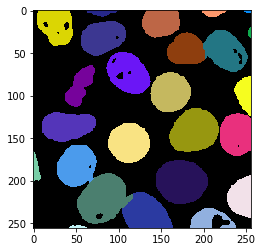
Seeded watershed#
We can use the image of labeled nuclei as starting point for flooding the low-intensity areas in the membrane image. This allows us to determine a cell segmentation.
labeled_cells = seeded_watershed(membrane_channel, labeled_nuclei)
imshow(labeled_cells, labels=True)
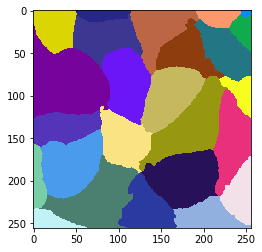
If the outlines of the cells are not 100% accurate, it may make sense to blur the membrane image a bit before segmenting the cells.
blurred = gaussian(membrane_channel, sigma=3)
labeled_cells = seeded_watershed(blurred, labeled_nuclei)
imshow(labeled_cells, labels=True)
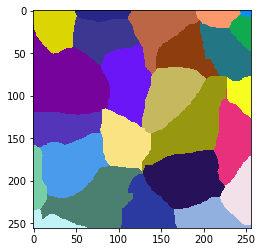
Seeded watershed using automatic seed detection#
In case we didn’t image a separate nuclei channel and only have the membrane channel available for segmentation, we can use the membrane image to search for local minima (dark areas).
labeles_cells2 = local_minima_seeded_watershed(membrane_channel)
imshow(labeles_cells2, labels=True)
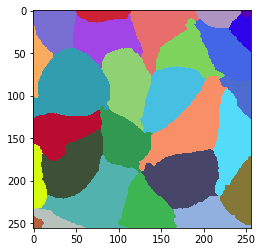
This function also has some parameters to allow fine tuning the segmentation. The parameter outline_sigma
allows to control a Gaussian blur filter that allows fine-tuning the outlines of the segmented cells as shown above.
labeles_cells3 = local_minima_seeded_watershed(membrane_channel, outline_sigma=3)
imshow(labeles_cells3, labels=True)
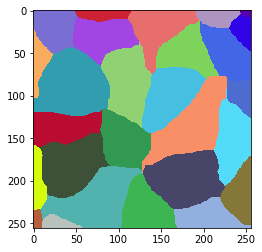
If there multiple cells sticking together, it may make sense to specify spot_sigma
. This parameter allows to configure how close / large cells are.
labeles_cells4 = local_minima_seeded_watershed(membrane_channel, spot_sigma=9, outline_sigma=3)
imshow(labeles_cells4, labels=True)
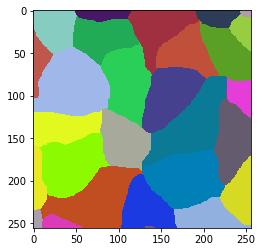
Exercise#
Load the following dataset and find good parameters for processing it using a seeded watershed approach. This example image data is a courtesy of Sascha M. Kuhn, Nadler Lab, MPI-CBG Dresden.
image_slice = imread("../../data/membrane_2d_timelapse.tif")[2]
imshow(image_slice)
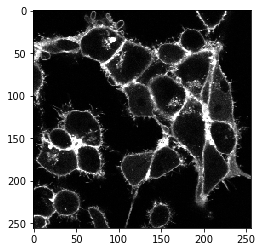